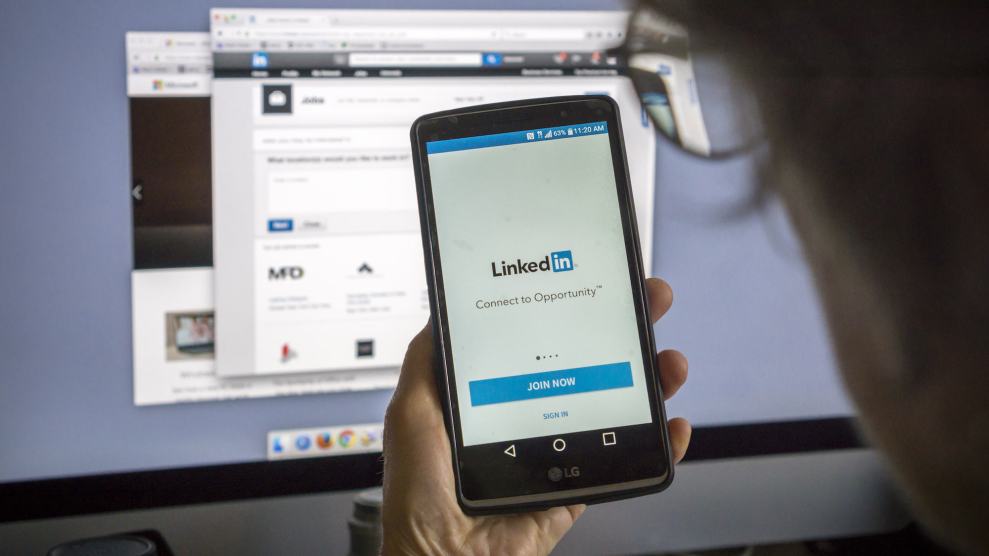
Richard B. Levine/Levine Roberts/Newscom/ZUMA
In September, ProPublica revealed that a number of high-profile tech companies were using Facebook to advertise jobs only to men. This seemingly clear violation of US employment laws would have been surprising had the news organization not found similar uses of the social-media platform’s advertising tools to discriminate against older workers in 2017. This finding led to Facebook being one of hundreds of parties named in a massive lawsuit for allegedly having discriminated against aging workers.
As a job advertising platform, Facebook uses what are known as predictive technologies to allow companies to preselect an audience for job listings posted to its site. This means that companies like Facebook and Google can preselect targeted groups for ads that platforms identify based on user data and behavior. In the past few years, automated hiring technologies have quickly overhauled the employment process at every step, offering employers services they say will cut through the human biases of the traditional hiring process. When a job is posted, companies like Mya and Montage often text candidates pre-interview assessments or allow for headhunters to swipe through on-demand interviews at their leisure. With artificial intelligence, other companies first analyze résumés for certain keywords that might indicate an applicant is a good fit for the job, then boost that application to the top of a recruiter’s virtual pile. Some companies, like HireVue and Predictim, have even promised to provide employers with AI-driven recommendations based on how a candidate smiles or speaks, a practice critics say relies on untested and potentially unethical science.
These technologies may promise to remove human bias from the hiring process, but they could actually be introducing more. According to a new report from Team Upturn, a Washington, DC-based public advocacy research group, the rapid growth of these technologies has outpaced understanding of them by both employers and regulators. The Equal Employment Opportunity Commission (which has seen delays in key staffing under Trump) hasn’t caught up, and the consequences for job seekers are significant. The report also found that predictive hiring tools can reflect systemic biases even at the same time they purport to ignore factors like race, gender, and other classes protected under the Civil Rights Act.
Mother Jones spoke with Miranda Bogen, a policy analyst with Team Upturn and co-author of the report, to learn more about how technology is introducing new opportunities for hiring discrimination, and what regulators need to do to prevent it.
Mother Jones: In the past, your work has focused on other issues of technological discrimination, including housing advertising and payday loan targeting. Why did you decide to study employment advertising technologies?
Miranda Bogen: When people think about hiring algorithms, there’s often this image in our mind that these tools are making “yes/no” decisions. It’s not only deciding who gets hired or not, or who gets an interview, but it’s also figuring out who has the opportunity to apply, who even knows about a job.
So we worked our way back to the beginning of the hiring process, which is what employers often refer to as sourcing. Because they’re getting so many applicants who find out about jobs over the internet, employers are now really incentivized to try to shape the pool of candidates even before people are applying. We find that prescreening tools, like where employers decide to advertise their job or even what tools they used to advertise, are becoming more popular and making all sorts of determinations about who should get shown a job opening and who should not. Sending in a job application presumes that you had the opportunity to send that application in the first place and that you haven’t been preemptively judged to not be a good fit.
Bias and discrimination have always been a problem in hiring decisions, so it’s easy to understand why employers are tempted by technology that seems to remove that opportunity by making the process more standardized. There are countless studies where, if you send in a résumé from different races or genders to the same employer, it’s clear the effect that cognitive bias has on the process. Vendors often talk about preventing those cognitive biases. But what they often aren’t grappling with is the fact that the replacement for the human cognitive bias is often more institutional and societal [bias].
MJ: How do technologies that are designed to eliminate biases actually introduce them?
MB: One of our big findings is that none of the tools we saw have sufficiently dealt with the underlying structural biases. So while these tools may be helping with one side of the equation, the human cognitive bias side, there’s certainly a risk of introducing these other kinds of deeper biases and doing so in a way that’s really hard to investigate and ultimately fix. The hiring process is so disaggregated now, there are so many steps, and some of these predictions and decisions are happening so early, it’s really hard to do that résumé-testing where we used to be able to understand the nature of the [discrimination] problem. Because predictive hiring tools that vendors are providing to employers tend to be opaque, candidates don’t even know they’re being judged by them, and employers might not really have great visibility into exactly what they’re doing. It’s really hard to get a sense of the state of the systemic biases and how much they really are being reflected in these tools despite attempts to remove them.
MJ: In November, the Washington Post wrote about an app called Predictim that purported to use AI to analyze a potential babysitter’s social media, and even their facial gestures, to provide a report about their “risk rating.” You were quoted as saying, “The pull of these technologies is very likely outpacing their actual capacity.” (Predictim halted services a few weeks after the Washington Post questioned its technology, and social-media platforms revoked the company’s access.) How does Predictim tie into the rest of your work?
MB: There are certainly questions about what data and signals are appropriate to use in hiring conversations. But a more fundamental question is, what data are companies relying on to decide who’s worth hiring in the first place? How did they define success internally? Are they clear on the weaknesses in that data, both in terms of whether their current workforce reflects historical bias, but also how difficult it is to actually figure out who’s a successful employee? A lot of executives already admit they have a hard time differentiating top performers from okay performers, and yet employers are basing predictive tools on this data that may or may not actually have anything to do with worker performance on the job.
Because the underlying data is so questionable, these tools that are scoring people on a scale of zero to 100 might be overstating differences between candidates who would actually perform equally as well. A recruiter who sees a tool that says one candidate is a 92 percent match and another candidate is a 95 percent match might be overly swayed by this prediction. In fact, it might not mean very much, but when it’s coming from a computer and when a number is attached to it, it can seem really compelling. These tools are often framed as decision aids for human recruiters who ultimately have the final say, but we just don’t know enough about how persuasive these tools are.
MJ: For people who are looking for things the EEOC or lawmakers could do to prevent or sanction this kind of discrimination, what are the unique challenges these technologies pose?
MB: The good thing is that anti-discrimination law is focused on the outcomes. It doesn’t matter if it’s technology or a human making biased decisions, employers are liable for discriminatory outcomes. The challenge here is that when we introduce technology into this process, the institutions that we’ve trusted to enforce these laws have a much harder time doing so because they might not have the capacity to do these sorts of investigations. The way that these laws have been interpreted by the industry and by the courts doesn’t fit with the capabilities these tools create.
For instance, some of the regulatory guidelines talk about how demonstrating that tools to assess job candidates are valid is if they can show that there’s a correlation between the thing that they’re measuring and some measure of workplace success. The point of predictive technology is to find correlations where we couldn’t normally find them. So we can draw correlations everywhere. But that doesn’t necessarily mean that the database tools we are relying on ought to be used to make hiring decisions. Under the law, these tools currently might be legitimate and employers might be convinced to adopt them, but the laws were written 30, 40 years ago and don’t grapple at all with the fact that so much more data is available than employers used to have access to.
MJ: Knowing this, are there any legal challenges that you’ve seen so far that could pave the way for regulation or reexamination?
MB: So far attention has been focused on the employers using these tools, but I think we are moving more in the direction of paying attention to the tools themselves. The American Civil Liberties Union brought a complaint before the EEOC about advertising on Facebook that did try to point to the role that Facebook itself as an advertising platform plays in creating the conditions where digital ads for jobs are being shown to certain people and not others. Our goal for the report is that in describing how these technologies are working in more specific detail, we could enable advocates to prevent discrimination from happening when these tools are in the mix.
Conversations about how the government should regulate technology and AI can get caught in a loop sometimes because it’s hard to articulate what the outcomes are that these laws are trying to shape or, for bad outcomes, they’re trying to prevent. With hiring, protections are built around outcomes. That creates a really strong framework to ultimately constrain what these technologies can do, as long as we have the capability to enforce those laws.
This interview has been edited for clarity and length.